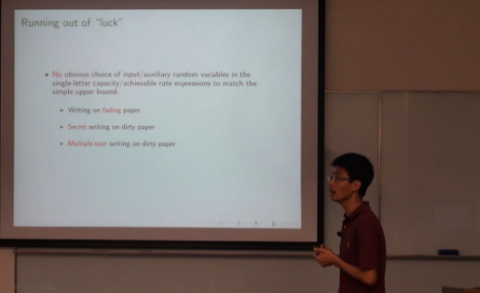
Recently there has been a lot of success in using the linear deterministic model (Avestimehr-Diggavi-Tse, 2011) to provide approximate characterization of Gaussian network capacity. This talk discusses the possibility of using the linear deterministic model to find approximate optimal input/auxiliary distributions for Gaussian networks. The viability of this approach is demonstrated via Gaussian channels with state and interference. A folklore in network information theory says that “Gaussian networks have Gaussian optimal solutions”. Interestingly, however, the approximately optimal distributions obtained via this approach are not necessarily Gaussian.
Tie Liu was born in Jilin, China in 1976. He received his B.S. (1998) and M.S. (2000) degrees, both in Electrical Engineering, from Tsinghua University, Beijing, China and a second M.S. degree in Mathematics (2004) and Ph.D. degree in Electrical and Computer Engineering (2006) from the University of Illinois at Urbana-Champaign. Since August 2006 he has been with Texas A&M University, where he is currently an Associate Professor with the Department of Electrical and Computer Engineering. His primary research interest is in understanding the fundamental limits of communication and cryptographic systems via the lens of information theory and leveraging these understanding into efficient engineering designs.
Dr. Liu received an M. E. Van Valkenburg Graduate Research Award (2006) from the University of Illinois at Urbana-Champaign and a Faculty Early Career Development (CAREER) Award (2009) from the National Science Foundation. He was a Technical Program Committee Co-Chair for the 2008 IEEE Global Communications Conference (GLOBECOM) and a General Co-Chair for the 2011 IEEE North American School of Information Theory. He currently serves as an Associate Editor for Shannon Theory for the IEEE Transactions on Information Theory.